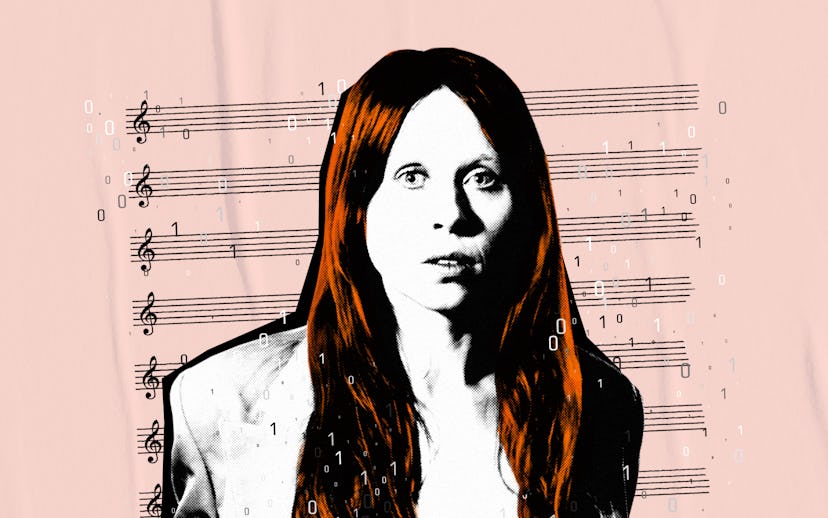
The New Pioneers
What Does The Future Of Artificial Intelligence In Music Actually Look Like?
AI music suggests a future of robot pop stars. But the reality is both less glamorous and less dystopian than that.
In late April 2020, a company named OpenAI uploaded dozens of new tracks to SoundCloud, all of them matter-of-factly titled like “Hip-hop, in the style of Nas” or “Pop, in the style of Katy Perry.” You’d be forgiven for initially thinking the songs were average YouTube covers. A few seconds spent listening to the gargled production, bizarre lyrics, and eerie vocals would definitely change your mind.
The songs were all made using an artificial intelligence software called Jukebox, designed by OpenAI, a billion-dollar research organization leading the field in AI research. Jukebox isn’t your standard Elvis impersonator: After being trained on 1.2 million songs and other data about genres and artists, the neural net has learned to produce original music in the uncannily recognizable style of famous artists like Elton John and Rihanna. It’s novel and impressive technology, a computer model that has figured out how to generate actual songs, including vocals, in raw audio.
When most people think of AI-generated music, something like Jukebox probably comes to mind — a sprawling architecture of code that’s mastering the art of imitation, creating music that could revive the careers of long-dead artists or create new ones out of zeros and ones. That imagery might explain why when OpenAI formally announced the tool in late April, Twitter reactions ranged from “This is amazing work!” to “this is both incredibly cool and deeply cursed” to the foreboding “The replacement of human intellect & creativity is surely arriving, brick after brick.”
“I find ‘artificial intelligence’... a bit obfuscating,” Holly Herndon says during a recent video chat from her Berlin apartment. No stranger to AI (the electronic and avant-pop musician’s most recent album, PROTO, was made with a nascent AI she named Spawn), Herndon thinks that the term’s loaded implications — of robot overlords and human obsolescence — mystifies its actual function in music. Most automated music creation happens through machine learning: training a model to analyze existing songs, identify patterns from the data, and use this knowledge to generate its own music. It’s a now-common process that hardly evokes the same sci-fi drama as "AI."
“Machine learning doesn’t sound as sexy [as AI],” Herndon says, “But it describes what’s happening. A machine is learning. And it’s learning from human intelligence.”
As it’s currently used in headlines and dystopian imaginings, "AI" carries with it a sensationalism that suggests every new development in music is bringing us closer to a future of robot pop stars. But the reality of AI music is both less glamorous and less dystopian than that. For one, it’s already here — existing in various ways, overseen by people with various end-goals, and, as Herndon points out, quietly learning from them how to behave. While the end results could be tech-dystopian, Herndon points out that nothing is set in stone; AI’s future in music is still being carved out, a path molded by the collaboration and conflict between any number of stakeholders. “I think what people fear with AI is not necessarily the technology,” Herndon muses, but rather “the hellscape society that human beings would build with that tech.”
When used as a vehicle for expanding human creativity, AI isn’t necessarily a threat. On PROTO, an album that pulses with experimentation yet remains steadfastly human-centered, Herndon weaves Spawn’s synthetic output with a sweeping choral ensemble to create moments of deep emotion. Blending the distinctly human and distinctly robotic into one, Herndon is both curating and directing the AI alongside the other bandmates; Spawn provides moments of creativity and innovative musicality, but Herndon is the one in control.
Musicians who experiment with AI are sometimes dismissed as gimmicky, despite legitimate artistic reasons for collaborating with the tech. When hackathon-team-turned-band DADABOTS placed second in the AI Song Contest that replaced Eurovision this year, they used an AI trained on a survey of 1950s acapella, pop, metal, and more. Other teams produced songs with machines trained on Australian wildlife sounds and texts taken from Reddit threads. DADABOTS member CJ Carr says that machine learning allows them to spin fantastical concepts and far-fetched inspiration into actual music. With AI, “our capability to collect, produce music, and collaborate with dozens or hundreds of artists expands,” Carr says.
But that doesn’t mean the technology is anywhere near creating (good) music on its own. Carr’s bandmate Zack Zukowski emphasizes how human intervention was critical for their success at AI Eurovision, saying, “We treated AI as if it was just another performer in our studio.” Incidentally, the team that let AI take the lead got last place. Even as the biggest recent breakthrough in automated music generation, Jukebox still has obvious limitations. It’s clear in the early samples that the tool hasn’t yet figured out chorus structures and often veers into distorted screaming. For now, there’s no comparison between human-made music and its AI-generated counterpart. Even if there was, our emotional attachment to the human elements of music suggests we’d be unlikely to give up music made by real-life people anytime soon.
"I think what people fear with AI is not necessarily the technology, [but rather] the hellscape society that human beings would build with that tech."
So why bother with AI music if we’re just pouring endless hours and billions of dollars into a machine that can only poorly mimic what humans have already figured out? Well, the answer depends on who you ask. For experimental musicians, AI is a way to make sounds like none heard before. While some stakeholders might be interested in churning out songs at the touch of a button, subsequently avoiding the cost of artist royalties. Others are driven purely by innovation for innovation’s sake, embodying the Facebook mantra of “move fast and break things.” Many more are still unconvinced that AI contributes anything good to what is largely considered an innately human art form.
For the optimists, AI has potential to fit into a narrative of music democratization. Stephen Phillips, CEO of Popgun, a startup with products that include an app that children can use to create songs with AI, is confident that more people being able to experiment with sound will only benefit music in the long run. “Our thesis has become that the biggest application of AI in music will not be to replace musicians but to make everybody feel like a musician,” Phillips says.
Technologies that help more people feel like they’re musicians, maybe even change the idea of who counts as a musician, have long pushed music forward, facilitating the birth of entire genres, from hip-hop to techno. It’s worth noting that those technologies, largely praised now as huge contributions to the industry, faced their own backlashes at the time of their introduction. Vocoders were accused of corrupting musical integrity, drum machines were deplored as a replacement for human drummers, and synths were disparaged as soulless.
Perhaps some of the similar resistance to AI music will fade as it gives way to understandings of new musical possibilities. Even early technology like the piano, Zukowski points out, “gave Mozart the ability to have quiet and loud notes,” expanding our understanding of what music could be.
Yet it would be deeply naive to suggest that humans only serve to benefit from this tech. Even as someone who is enthusiastic about AI music, Herndon is on edge about AI’s serious potential to hurt the very artists whose discographies it trains on, the musicians who effectively made the machine what it is. She found Jukebox’s focus on impersonation alarming, to the point that she contacted the OpenAI team with her concerns. “It's a very entitled approach to other people's personhood and data,” Herndon tells me, “to take an artist's likeness, to train on that, and then release things in someone else's image without their permission.”
Herndon’s issue with Jukebox involves a tricky question of intellectual property that has plagued AI music from the very beginning (as well as sampling before it). While the right to fair use isn’t to be restricted lightly, Herndon emphasizes that the evolving field of AI music law will need to account for the systemic flaws that influence both our algorithms and the humans controlling them. Given tech’s much-discussed problems of racial bias and the music industry’s well-documented history of underpaying Black musicians, the likelihood of a racialized effect from AI music is not insubstantial. Herndon fears that AI music could produce a “punching down” rather than “punching up” effect where big companies reap the benefits of lax intellectual property laws while independent musicians go unpaid and unrecognized.
“All technology gives people power … and this power can be given to artists. I'm taking this technology and putting it in the hands of producers that have something to express.”
Something of the sort may be happening in the fields that AI has already penetrated. Michael Donaldson, who owns a music licensing company, tells me that in his industry, production music — the background songs that content creators license for videos, podcasts, and other media — is increasingly a breeding ground for AI development. Since most production music is already tailored to creator-friendly metrics such as “happy” or “corporate,” the human product is already done algorithmically. “Anything that can be made to spec can eventually be done by a computer,” Donaldson says.
Though production music tends to be written off as generic, uncreative work, it’s nonetheless a lucrative field that provides work for many professional musicians. But given how AI generates production music much quicker than humans, and can seemingly do it as well, an eventual takeover isn’t unfathomable. “That industry is dead,” Phillips predicts, if AI continues its hold. It’s not a stretch to imagine the tech eventually spreading into other areas of music creation: film scores and chart toppers.
But if there’s an argument refuting the possibility of an AI takeover, its most convincing tenet might be this: Humans like music because other humans are making it. Our capacity to relate to each other, to know what speaks to each other in music, is something that AI isn’t even close to figuring out. “It doesn't know how, you know, that one song just hits that summer,” Herndon says. “That takes a human brain and ears.”
For now, the artists who work with machine learning are choosing to focus on how they can use this technology to augment rather than replace their own creative projects. Herndon’s next project involves a serious Spawn upgrade. DADABOTS is launching an initiative against police brutality using Jukebox to generate hundreds of versions of N.W.A.’s “[expletive] tha Police” in different genres. They’ll curate the best 100 for free release and host a remix contest, a musical protest intended to help those with something to say find new ways to say it.
“All technology gives people power … and this power can be given to artists,” Carr says. “So we're taking it. I'm taking this technology and putting it in the hands of producers that have something to express.” Used like this, AI enables new collaborations and amplifies new voices, the very things that make music great. And the idea to do such a thing, to send such a message, in the first place? That’s a wholly human endeavor.
This article was originally published on